Other Kinds of Inference
cross posted on: https://crispychicken.cc/2021/02/09/other-kinds-of-inference/
In 1822 some people in what is now Germany were like: "Yo, isn't that an African arrow in that stork's neck?"

Sure enough it was, and this lead to a lot of progress on the question of "Where the hell do birds go when they're not here, anyway?"
All in all it was a great day.
One thing that I love about this event, is that it's pretty hard to explain the reasoning that gets you from "African arrow in a stork" to "migration" as scientific in the overly-narrow definition given to "the Scientific Method". Of course, a lot of other things made migration make sense, but fundamentally the reasoning process that went into this wasn't statistical inference.
When I tell this to my fine colleague Suspended, he tells he "idgi" and "can't we just describe that as Bayesian reasoning?"
Foolishness!
Am I'm just fetishizing the idea that we can wrest some ground back from "falsification radicalists" who want science to be about t-tests and nothing else? No.
The devil, as usual, is hiding in the details. I really have no objection to the statement "we could invent a Bayesian system to describe this inference" or "maybe the brain is all just Bayesian inference." Maybe it is! The problem is that I believe theories because of their instrumental value, and this kind of Bayesian description always seems to happen after the fact. The kinds of models Bayesians I know make are so general I wouldn't know how to begin applying them, or else so specific it's not clear how thinking can really be reduced to them.
And I think I know why.
Science, statistics, and the quantitative crowd as a whole has very little respect for the fact that the representation and organization of knowledge is often the key to the entire thing. It doesn't matter if all you do is memorize, as long as your internal archive is structured in such a way that you can recall the precisely needed fact in the right context. Indeed, this seems to be much of what learning actually is; consider the last time you tried to learn a language.
But current attempts to explain reasoning processes as statistical invariably assume a representation to do statistics over. To me, this is like explaining how a car works by assuming that you already have a working engine and you just need to connect it to the axels. Something is happening at the heart of this system to create and maintain a map of the world while doing a million other things, so assuming "P(birds go to Africa sometimes | arrow in stork) is close to 1" is just something that the brain can pull out if it wants to is ridiculous. It's downright ridonculous.
This gets down to the fact that quants usually believe the brain is capable of a kind of hyper-symbolic calculus that will discretely represent anything that's useful. The brain is eventually capable of such symbolic reasoning—but it must become acculturated to it. When people learn calculus they struggle a lot with infinitesimals because they need to get used to this new subset of symbols and how they're manipulated. This tells us our brain is good at reasoning symbolically in domains, and the history of innovation tells us that at least some people are really good at transferring over patterns from one domain to another.
But how does this transferring over work? It's not statistical inference, but a kind of "reasoning by models". Consider this classic XKCD comic:
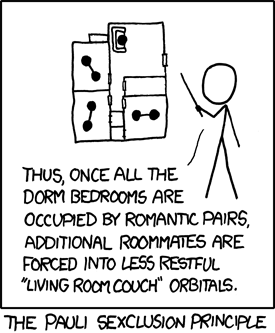
This is another kind of reasoning, it's one where you choose to think about two phenomena that share certain properties as possibly sharing more properties than the ones you've already observed: analogical reasoning.
But there's no really semantic difference between reasoning, inference, and significance testing, only a few details about application. Statistical significance tells us when a result is likely not caused by the kind of randomness we're assuming would be the default in a given scenario. But it's just as reasonable to consider "axiomatic significance"—where you start with a number of axioms and, upon observing something that breaks your system, start to look for which axioms would need to be removed to fit your theory.
What we see in Pfeilstorch—wherein people stopped believing the birds turned into mice when they vanished from the trees—is a yet more complicated kind of reasoning we have studied very little...until Cognitive Science and Machine Learning have simultaneously begun to force us to acknowledge that intelligence rests on inherently shaky (and therefore flexible!) ground: distributional simulation. Migration is a theory that was put together under the understanding that there are certain things that animals are simply more likely to do (like move) than others (like turn into mice, or die only to appear next year). The space of "things that could happen" was limited to the kind of stories people were willing to construct, and turning into mice was something people had thought about a lot in fairy tales before. But without the creation of these kinds of intuitive theories statistical inference has nothing to falsify.
But it's more than just a foundation for statistics. Much of the time you don't need any explicit statistics at all, because you're not working with enough explicit data that you could ever make a real argument one way or the other. Instead, you need to reason under the dynamics you can agree on with your community. It sounds ridiculous, like a lesson in management but it's the original kind of inference. If you wanted to avoid lions, you had to agree with your fellow hunters where to go under some understanding of lion dynamics and how they refer to lion observations.
If you think we've gotten past that I challenge you to find another basis for the World Happiness Report. On what other basis than agreed dynamics could we ever discuss hypotheses about human behavior? Most sciences are not predictive, it is time we started explaining on what foundations they stand, instead of being mildly embarrassed whenever the topic is brought-up.
Linked from: